Machines are an extension of human ingenuity, intelligence and creativity; they can accomplish tasks that are otherwise redundant, difficult or an inefficient usage of time for humans to complete. Artificial intelligence (AI ) was first achieved through the creation of neural networks, based loosely on biological neurons; AI became an actively pursued field of study in the 1940s. However, as machines’ capabilities increased, so did the implications surrounding them. Modern day AI models such as text-to-image generative models, natural language processing models, and many more are usually trained utilizing data scraped from the internet. Billions of image-text pairs utilized specifically for the AI technologies are now becoming available to the mainstream public, without a conversation on their ethicality, and most importantly, the biases surrounding the data. My research will focus on the visual biases present in text-to-image models. My work illustrates the inherent biases present within data sets, with biases distinguished either as the public’s bias (formed through Stable Diffusion’s data), or the private (formed through collected data of my own, and of those around me). I ask: How does the visual bias present in an image refer to the biases of the general public? How does visual bias allow for a representation of the personal biases present? And what happens when all these biases are merged together?
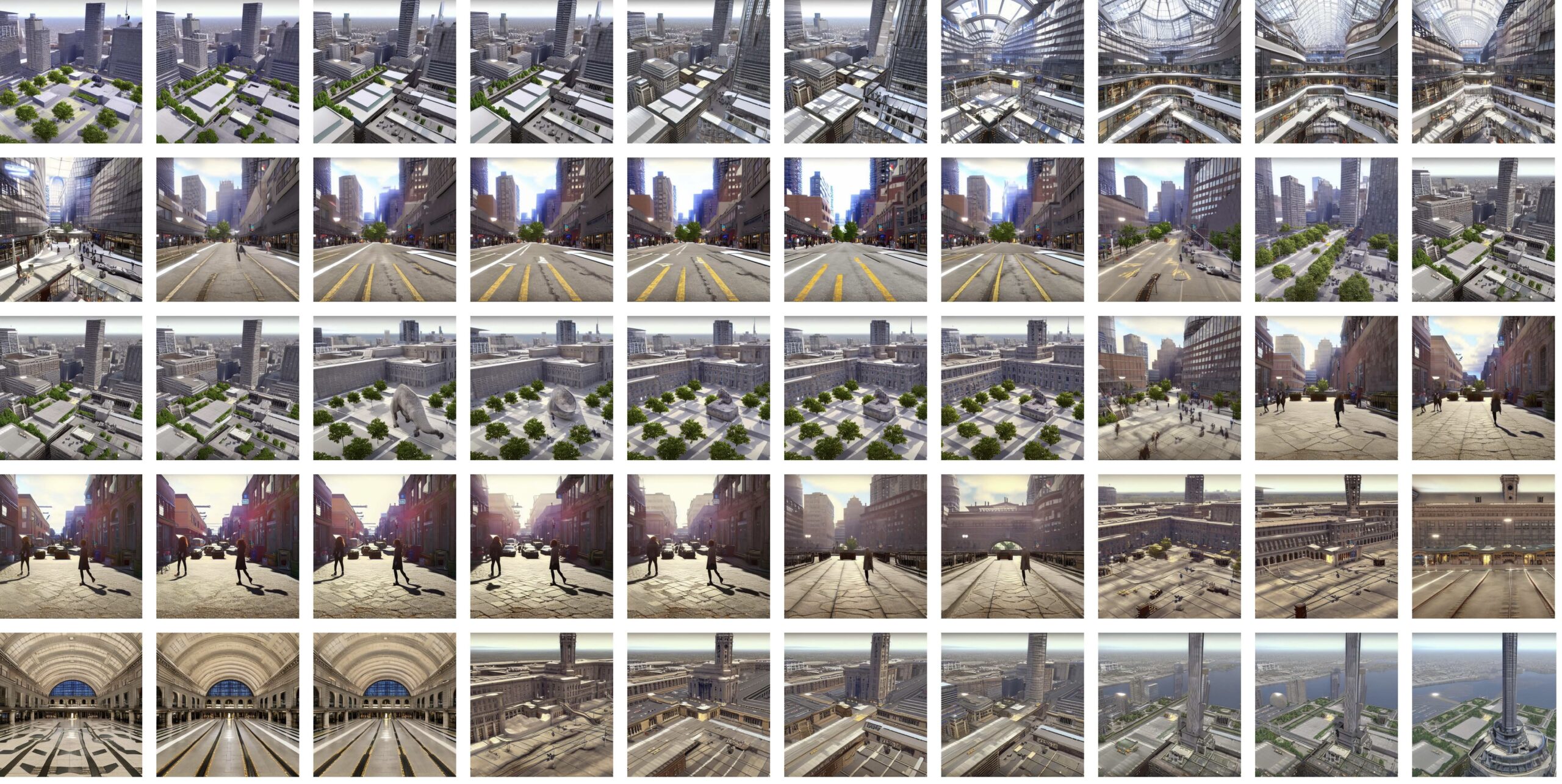